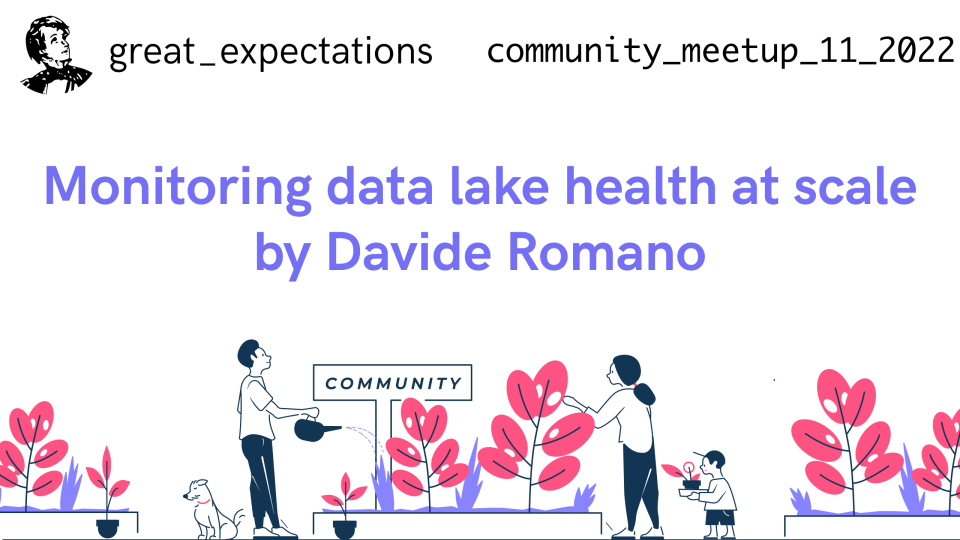
We get the community together on the third Tuesday of every month.
At meetups, we discuss the Great Expectations roadmap, watch ecosystem integration demos, and explore different ways data leaders have implemented Great Expectations. Sign up here to join the next one!
Now, let's dive into the roundup.
We covered
A Great Expectations product roadmap update
Welcoming GX’s new Director of Developer Relations, Josh Zheng
GX Airflow operator improvements
Monitoring data lake health at scale, from Davide Romano
Roadmap update
Recently: Data Assistants launched on September 20
The first Data Assistant is the Onboarding Assistant. Data Assistants replace the UserConfigurable Profiler. They’re new, so be sure to give us your feedback!
Current focus: Making it easier to get started
In terms of features, we’re working on:
Improving DataContexts with better doc strings and more consistent save behavior.
A new approach to data sources. Watch an overview in last month’s update.
We’re also improving the developer experience:
We have new goals for responding to contributor PRs, and aim to respond within 24 hours and merge within a week (depending on scale). An important note on this: if we close out your PR due to inactivity, don’t hesitate to reopen it when you’re next able to continue the conversation!
We’re also focusing on creating API documentation that’s on par with other API docs that we love, like Pandas’ and SciPy’s.
Up next: SDKs
Improved API docs, automated tests and test environments, a well-defined publishing experience, and more.
Watch the roadmap presentation:
Welcome
We are thrilled to welcome Josh Zheng, GX’s new Director of Developer Relations!
Josh is excited to meet community members—don’t hesitate to DM him @Josh Zheng on the GX Slack, and if you’re in the Bay Area, he’d love to buy you a coffee and talk GX in person.
Feature update
Exciting news about the Airflow operator: in collaboration with the Astronomer team, we’ve officially turned ownership of GX’s Airflow operator repository over to the Astronomer team for long-term maintenance with their other operators.
An announcement is forthcoming about a new version, but the updates will make it easier for new GX users and add improved support for OpenLineage.
Check out the video for additional comments from Benji Lampel, Airflow Engineering Advocate at Astronomer.
We appreciate the Astronomer team’s commitment to ensuring a best-in-class integration with GX!
Community demo
This month we had a great presentation from Davide Romano, a data scientist at Mediaset.
Watch the presentation here:
Or read on for a summary!
Background
Mediaset is a leading private TV publisher in Italy and Spain that built a data lake with 100GB of incoming data daily. As data volumes and daily queries increased, Mediaset advanced to actively ensuring their data lake health. They used Great Expectations and Spark to build a data quality framework.
Needs
Mediaset had four critical data quality needs:
App event tracking verification
ETL pipeline unit tests extension
Pipeline and services monitoring
Data drift detection
Workflow
Mediaset has a five-step workflow:
A Docker image running a Jupyter notebook and GX is used to develop Expectation Suites, including Custom Expectations. Custom Expectations are key to Mediaset’s GX implementation.
Once complete, Expectation Suites are committed to Mediaset’s git repository via a CI pipeline. The Expectation Suites, Custom Expectations, and Data Docs are stored in S3.
Airflow executes the data quality DAG.
Apache Superset is used to visualize results and send alerts.
GX’s Data Docs are published in Mediaset’s data documentation. This is key for sharing table checks with Mediaset’s nontechnical team members.
Mediaset’s data quality evaluation process
Davide explained how Mediaset incorporates insight from its nontechnical domain experts with a feedback loop between domain experts and GX experts to fully define the data quality requirements before GX is used.
Davide also answered a few questions from the audience. Click here to skip to the bonus/Q&A portion of the talk:
And the conversation continued in the GX Slack.
Thank you Davide for sharing your experience!
Read Davide’s article on Medium, and see the hands-on repo at Github.
Join the data quality conversation
davor korman had a question about keeping Data Docs from being overwritten on S3.
Gabriel Ferreira shared his chat with Martin Sahlen about why data lineage is hard.
Heather looked for advice about using batch_filter_params with split_on_year_and_month_and_day.
Brendan Bull asked about improving cluster utilization during concurrent Validations on Databricks: weigh in at Github!
Antonio Calvacante wrote a blog post about using GX to validate Delta Lake tables.
Additional updates
Get the invite for December’s meetup here.
GX now has CLI support for Trino (thanks, hovaesco!), plus some additional bug fixes and doc improvements in the latest release, v0.15.32.
Check out our tips on using Boto3 to Assume Role with AWS, passing Spark configuration parameters, and specifying nonstandard delimiters for your CSV files.
We’re hiring in product and engineering! Check out our open roles.
Have you done something cool with Great Expectations that you'd like to share? If you're interested in demoing or have a piece of data quality content that you'd like us to feature, DM @Kyle Eaton on our Slack.