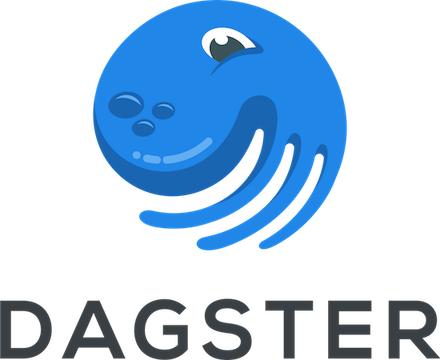
We’re really excited to announce an initial release of a collaboration with a fellow open source project - Dagster. As of version 0.9.3, Dagster now includes Great Expectations rendering right in its development tool, dagit. If you’re also excited now, you probably already know enough about Dagster and Great Expectations and can jump right into the Dagster documentation about the integration. If not, read the post below to learn more!
What is Dagster?
Dagster is a data orchestrator for machine learning, analytics, and ETL that allows you to define your pipelines in terms of the data flow between reusable, logical components. You can implement components in any tool, such as Pandas, Spark, SQL, or DBT. Whether you’re an individual data practitioner or building a platform to support diverse teams, Dagster supports your entire dev and deploy cycle with a unified view of data pipelines and assets.
How does Dagster integrate with Great Expectations?
Dagster's graphical frontend, dagit, is designed as an environment for local development and production operations. It can also be run as a production service, to support operating, debugging, and maintaining large-scale production data pipelines. The Dagster team collaborated with the Great Expectations core engineering team to integrate Great Expectations validation rendering right into the dagit UI. This allows you to create a simple Dagster solid (a node in the DAG), in which you can create a Great Expectations data context, get a batch of data, and run validation in just a few lines of code, as well as inspect the validation results directly in the dagit UI.
Why are we so excited about this integration?
Data quality is a huge part of your data pipelines - we’d even go as far as to say that your pipelines are pretty much useless if you don’t have a way to verify that the data you’re pushing through those pipelines is actually correct. Great Expectations allows you to specify exactly what you expect the data to look like at each point in your pipeline, so that any unexpected changes can be spotted quickly.
Integrating a data quality tool such as Great Expectations into dagit means we’re combining two powerful tools into one UI, which makes it even easier to get serious about data quality and data testing. Plus, we’re also just really jazzed about a successful collaboration with an awesome engineering team working on another open source project!
Where can I find more information?
Check out the Dagster github page here
Read the Dagster team’s blog post about the integration with Great Expectations