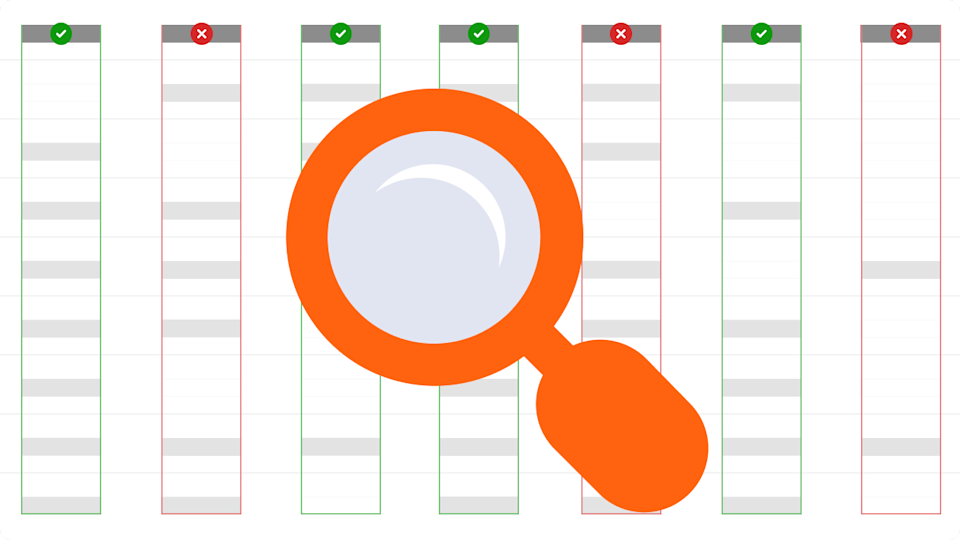
This post is the second in a seven-part series that uses a regulatory lens to explore how different dimensions of data quality can impact organizations—and how GX can help mitigate them. Read part 1 here.
Belonging to a data team isn’t just about managing information. It’s about making sure everyone in your company can make fully informed decisions. Ultimately, your work shapes the strategic direction of your organization.
In this blog post, we’ll explore how missing data—an obvious issue, but one with more subtleties than you might expect—can negatively impact your data… and what you can do about it.
As we delve into the world of missing data, we’ll once again use the lens of FinTrust Bank, a fictional financial institution. We’ll explore how a high degree of data missingness can impact risk assessment and regulatory compliance, and how you can effectively use GX to manage and mitigate the challenges of missing data.
A pervasive challenge
Perhaps unsurprisingly, data missingness is a widespread issue affecting organizations across essentially every sector.
In some ways it might seem to be a relatively mundane data quality issue—it doesn’t take a deep technical background to spot an empty field—but that doesn’t mean it’s low-stakes. In healthcare, missing patient data can impact treatment decisions; in manufacturing, gaps in production data can lead to inefficiencies, health and safety issues, or low-quality products.
And in the financial sector, missing financial data can lead to off-base risk assessments, operational inefficiency, and more.
For an example, we return to FinTrust Bank, where their recent data audit has discovered that key data was often missing from datasets. Specifically, critical fields in loan application data are routinely incomplete.
The ripple effects of missing data
The implications of missing data extend throughout FinTrust Bank:
Skewed analytics due to incomplete data can lead to biased analyses and flawed decision making.
Regulatory misreporting because of omitted data can result in penalties and increased scrutiny.
Operational inefficiencies happen when remediation is dependent on ad-hoc manual intervention: employees spend time on repairing data instead of their main job, slowing down the bank’s processes and creating an opportunity for human error to introduce a new quality issue.
Missed opportunities from incorrect risk analysis and misclassified opportunities.
Leveraging GX to tackle missing data
Samantha, the data engineer leading the quality improvement effort at FinTrust Bank, chose GX as their data quality solution. That means she has access to its robust tools for detecting and quantifying missing data, so she can quickly build a comprehensive understanding of their data’s completeness.
With GX Cloud, Samantha’s team collaborated with each other and with subject experts outside of the team to develop and refine Expectations that built a meaningful understanding of their data’s expected completeness.
That included identifying where missing data is actually a problem, identifying the degree of missingness that warrants remediation, and setting up alerts to notify the appropriate stakeholders immediately when there’s a missing data incident.
With GX Cloud’s easy-to-use SaaS interface, FinTrust Bank team members could work simultaneously, examine results, and track changes in real time. This significantly accelerated their data quality work.
Key Expectations for missing data
Samantha’s team quickly implemented the key Expectations for missing data. They used ‘Expect column values to be null’ to check for the presence of null values in critical fields like loan amounts and application dates, where any amount of missing data is unacceptable.
Other fields, like applicant credit score, required more nuance. On occasion, an applicant is applying for credit for the first time, and has no credit score. This is unusual but not unheard of, so Samantha’s team set a threshold allowing up to 1% of credit score values to be null.
By allowing this small degree of flexibility, FinTrust Bank stakeholders won’t be unnecessarily alerted every time a first-time credit-seeker applies—but they will be notified if the amount of missing data reaches unexpected levels, indicating a true missing-data problem.
After applying missingness data quality checks across FinTrust Bank’s data, Samantha’s team uncovered some crucial insights about their data completeness. They found that 3% of loan amounts were missing, 5% of credit scores unavailable, and 7% of employment history records incomplete.
Strategies for addressing missing data
With insights from GX, Samantha’s team developed a comprehensive strategy to tackle their data gaps:
They redesigned the loan application form, which dramatically reduced missing employment histories.
They applied statistical techniques to fill loan amount gaps while maintaining data integrity, flagging all imputed values for transparency.
They standardized the definition of ‘missing’ data across departments, ensuring consistent identification and handling of the issue.
They automated their data quality checks using GX Cloud, ensuring that future errors could be identified quickly using a transparent set of criteria.
They conducted root cause analyses to improve their other data collection strategies and prevent future data gaps.
Finally, they integrated the automated alerts that they had set up in GX with their team communication tools. When critical data goes missing in the future, the right people will now find out immediately.
Outcomes and impacts
FinTrust Bank saw immediate results from their improved data missingness checks. The overall rate of missing data dropped by 60%, while the time spent on manual data cleaning decreased by 40%. Perhaps most importantly, the accuracy of their risk assessment models improved by 25%.
As an example of improved outcomes, the higher-quality data was used to assess a high-value commercial loan application. The data indicated that FinTrust Bank could offer competitive terms while maintaining appropriate risk management, allowing them to secure a $10m deal.
Later, the original, pre-GX data was used to reproduce the assessment process on the loan application. Samantha’s team found that the old data’s conclusion would have led FinTrust Bank to pass on the opportunity.
Elevating data quality: a strategic imperative for competitive advantage
Tackling missing data isn’t just about compliance. It’s a strategic imperative that can transform your organization’s decision-making capabilities. FinTrust Bank’s example shows that with the right tools and approach, significant improvements are within reach:
More accurate risk assessment
Better decision making
Increased operational efficiency
Enhanced regulatory compliance
As your data quality work continues, you’ll continue to evolve your understanding of how and when missing data most affects your organization. GX Cloud makes it easy to reflect those changes in your tests, so your Expectations stay a reliable central resource about how your organization handles missing data.
Join the discussion
How could better handling of missing data benefit your organization? In our community forum, you can share ideas and experiences with other data practitioners. Together, we can improve best practices for implementing a data quality process.
Stay tuned for part 3 of this series, where we’ll look at monitoring your data volumes.