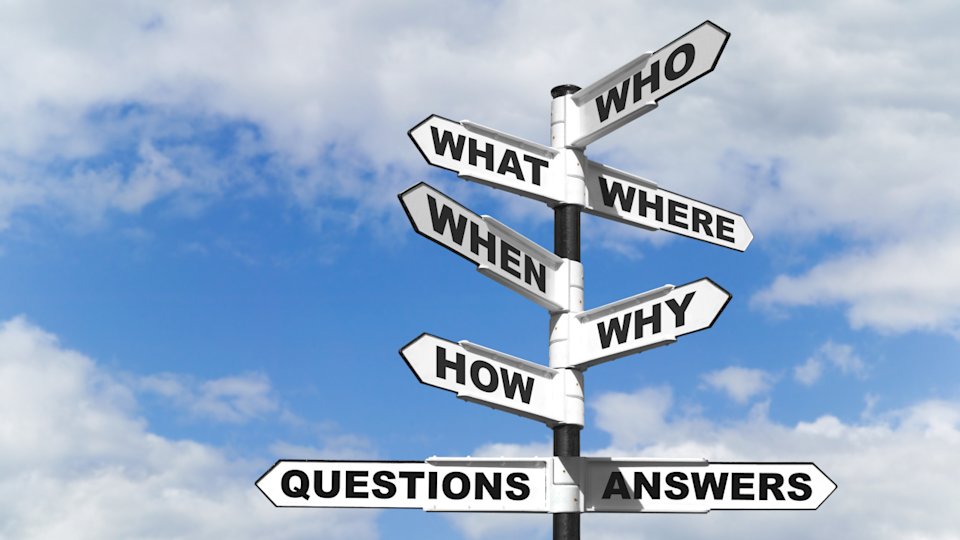
Never mind what data quality means—is data quality a tangible thing? A state of being? An activity or maybe an adjective? Working with data is all about precision, so a nebulous “data quality” concept doesn’t sit well.
In this post, we’re going to refer to “data quality” as a “data quality process.” And what we mean by that is:
A data quality process is an iterative, ongoing process ensuring 1) that you know whether your data meets the expectations and standards you have for it, 2) that all members of your organization know what those expectations and standards are, and 3) that those expectations and standards meet the current business and operational needs of the organization now and in the future.
Snappy? No. Precise? Yes. And that’s what we’re going for.
Where to start
A data quality process has three major components:
The technical implementation (ensuring that you know whether your data meets the expectations and standards you have for it)
Communicating the shared understanding (ensuring that all members of your organization know what those expectations and standards are)
Establishing the shared understanding and keeping it updated (ensuring that those expectations and standards meet the current business and operational needs of the organization now and in the future.)
When someone says “data quality,” the technical implementation is probably what you think of first, which is why we put it first in the definition.
But definition isn’t practice, and you shouldn’t start by implementing anything.
Instead, building a sustainable data quality process looks like:
Establishing a shared understanding
Technical implementation
Communicating the shared understanding
Updating the shared understanding
Text isn’t the ideal medium here because a data quality process isn’t linear:
Your data and requirements will change, which means your process will have to change too.
If you’ve implemented your data quality process the right way, you’ll have already established a successful, tested, and proven strategy for iterating on it.
But you do have to start somewhere. Here’s how to figure out where.
Choose a direction
Almost as soon as you start building your data quality process, it’s very easy to be pulled in a lot of different directions.
To make the best use of your resources, it’s good to know what kinds of activities are your highest priority. Some questions to ask yourself:
Is breadth of coverage (number of data sources tested) or depth of coverage (tests per data source) most important to me?
What are the points in my pipeline I’ve had problems with in the past?
Is my data quality process implementation being motivated by a specific outcome? If so, what would contribute to that outcome?
Pick a starting point
The journey of a thousand data quality checks begins with a single set.
Now that you know your general priorities, what data source are you going to start with? It’s possible that the questions you answered in “Choose a direction” above have answered that. If so, skip ahead to “Gather your team.”
But if you’re still having trouble narrowing down the options, see how many of these points are true for each of your top contenders:
I don’t control the source of this data
Customers see this data
Partner organizations see this data
Issues with this data can have regulatory implications
This data is part of my organization’s core function
I know at least three people or teams who use this data directly
We’ve had problems with this data in the past
The more of these boxes a data source checks, the higher you can move it on your list.
Strategic considerations
An important factor to consider when choosing your data sources is strategy.
In general, we recommend picking a data source that’s used frequently and is of moderate or high importance to the business. This makes it easier to get buy-in, attention, and effort from stakeholders—and you’re going to need that.
It’s doubly important to think strategically if you’re trying to make a case for getting or increasing resources for your data quality process.
Being able to point to quantitative results is key for convincing skeptics. So, is there a prominent dashboard that regularly breaks or a customer who frequently complains? Focusing on the data sources behind a high-profile issue will let you quickly begin accumulating evidence for why your data quality process is worth investing in.
This kind of strategy isn’t necessarily driven by technical considerations, so it’s easy for the technically-minded to overlook it. But to set up your data quality process for long-term viability, you really shouldn’t.
Gather your team
No one is an island, so your data quality process has stakeholders. And those stakeholders have different degrees of technical comfort… but you still need them to be able to contribute to and comprehend the shared understanding of the data.
In general, you can divide stakeholders into technical and nontechnical groups.
Technical stakeholders include people in roles similar to yours. They also include software engineers, application maintainers, and anyone who handles the infrastructure around moving or generating your data.
Nontechnical stakeholders are everyone else.
Anyone who uses the data or a product of the data (like a dashboard, analysis, or report) is a nontechnical stakeholder. So is anyone who owns a relationship with an external stakeholder: sales representatives are stakeholders for data that appears on customer-facing dashboards, for instance.
Obviously, involving all stakeholders personally in your data quality process is a non-starter. In practice, you’ll typically have a representative stakeholder for a group: perhaps all of customer service is represented by one of the customer service managers.
Since a data quality process is iterative, start small. Pick a couple of key stakeholders to engage immediately, making sure at least one of them is nontechnical. Your initial data quality process implementation can produce value with as few as 2-3 stakeholders directly involved.
Conclusion
When you’re working with something as important as your data, it’s vital to build on a strong foundation. And “data quality” without further specifics is way too vague to support anything you want to last.
In this blog, we’ve gotten rid of the nebulous references to “data quality” and established a clear definition of a data quality process:
A data quality process is an iterative, ongoing process ensuring 1) that you know whether your data meets the expectations and standards you have for it, 2) that all members of your organization know what those expectations and standards are, and 3) that those expectations and standards meet the current business and operational needs of the organization now and in the future.
And if you’ve put all our tips above into practice, you’ve also identified your top priorities, a data source to start with, and a handful of stakeholders who represent diverse perspectives.
Put this all together, and it creates the bedrock you need for a data quality process that gets things done.
GX Cloud provides an end-to-end solution for building and managing your data quality process. It's a fully managed SaaS solution that provides an intuitive interface for the world's most popular data quality framework. Sign up and see for yourself, free.