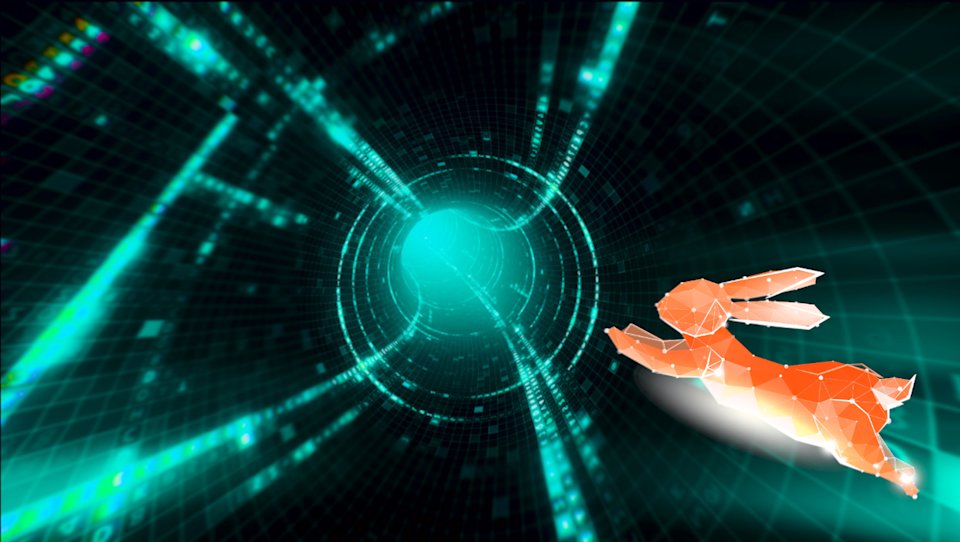
The world is full of things that feel like they should be solved problems but aren’t. Like not getting reminders to schedule appointments you already scheduled or stopping mail addressed to the person who lived in your house ten years ago.
Consistently having high-quality data that you can confidently use for its intended purpose is one of those things.
Why are dashboards constantly breaking, why are reports missing information, why are the analytics always late? We can generate all this data automatically, but trying to do anything with it takes so much manual effort.
Why don’t we have the tools to solve this universal problem?
That’s not the right question.
Let’s back up a minute. You’re trying to do something with your data. You want to see what users are doing in your app or whether your machine learning model acts as you expected. What ads are most effective with which customer segments? Is your application meeting your uptime SLAs? How much money did your company make last quarter? Do you have everything you need for your regulatory reporting?
Whatever you’re using your data for, the basic reason you’re using it is that you want to learn something.
If you already had the knowledge you were looking for, you wouldn’t need to learn it. So, by definition, you are going to be surprised by what the data says.
(Is your level of surprise going to be more “aha” than “shocked and astounded”? Yes, maybe. But the situation is the same regardless.)
The pivotal question is: why are you being surprised? There are two possibilities:
You're being misinformed about the state of the world because the data has an issue.
There is new information about the state of the world, which the data has revealed to you.
You can see the informational asymmetry: to learn, you have to be surprised. But you can be surprised and not learn.
To know that you’re learning about your app, your ML model, your ads, your uptime, your income… you have to be confident that nothing went wrong while collecting or processing your data.
And maintaining confidence in your data collection and processing hinges on how you deal with those surprises. The key is:
Understanding the quality of your data isn’t the process of finding the right answer; it’s the process of ruling out all the wrong answers. Which takes much longer because there’s no possibility you can stop early.
And you probably aren’t going to be able to stop after one round of it, either. You need confidence in the data that you’re being surprised by right now, yes, but underlying it is more data that also came from somewhere, and under that is yet more data…
The higher the stakes, the deeper the rabbit hole. Following it all the way down is a lot of work.
It would be bad enough if it were a lot of work that you could do yourself. But to get full context and understanding of your data, where it comes from, how it’s used, and why, you need the collective knowledge of everyone who works with or uses the data. For high-stakes, high-usage data, that could be dozens of people (or even more) for a single dataset, let alone the entire pipeline behind it.
That's not just a lot of work; it also requires a lot of people.
And a lot of people means infinitely more opportunities for miscommunication, or for completely conflicting perspectives on exactly the same data (neither of which is wrong, exactly), or for someone to leave and take crucial knowledge with them.
At the risk of pushing the metaphor too far, the rabbit hole is actually a full-on rabbit warren.
So, is it hopeless? Absolutely not.
We didn’t say all that to make you feel depressed or overwhelmed. We said it to put the right lens on data quality.
The issue isn’t: we don’t have the tools. It’s: we haven’t been putting the tools together in the right ways yet.
Because the technological problem in data quality isn’t pure computation. It’s just as much, if not more, about communication. Evolving, collaborative, co-co-co-co-...authored communication that evolves over time without losing history.
Communication that’s fully, totally centralized, so everyone sees the same thing no matter where they are in the rabbit tunnels.
Knowing that, it’s easy to see why data quality isn’t ‘solved’—high-quality data will always be a moving target. Ensuring high-quality data means building a durable process.
Nothing turnkey can create an effective data quality assurance process with a button press (or even a series of button presses). Because having confidence in the quality of your data needs more than the right software tools. It requires insight and investigation and communication and cooperation, from the specific people at your specific organization who work with your specific data.
And now that we understand the real challenge, we’re empowered to make real progress with developing data confidence.
We can pick the right tools, bring in the right people, and build the right processes for handling all the surprises waiting in our data.
GX Cloud from Great Expectations helps you build confidence in your data with a friendly SaaS interface and plain-language tests and results, so it's easy for data teams and stakeholders to communicate and collaborate: try it today.