Have confidence in your data
Get started quickly
Use GX OSS with tools you’re already familiar with, like Python and Jupyter notebook, as part of its developer-first experience. Its minimal technical prerequisites mean maximum readiness for use.
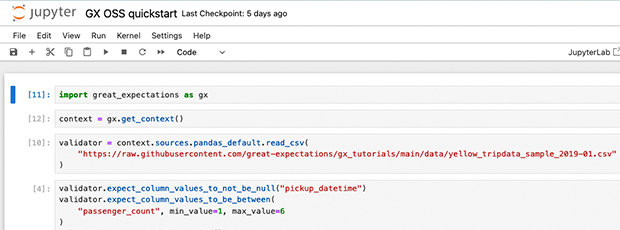
Create a shared point of view
Working with other technical teams can get complicated when everyone’s using different languages and approaching the same issues from different perspectives. Using GX OSS gives everyone a shared toolset and starting point while still allowing each team their own flexibility and independence.
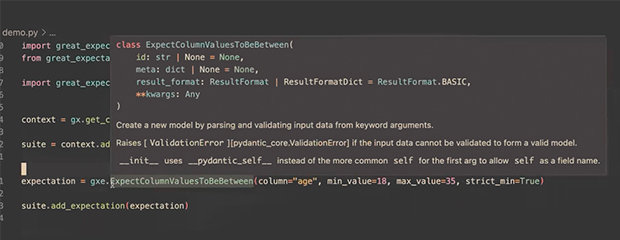
Communicate better
Stakeholder input is key to making sure your data quality meets the needs of—and is embraced by—the organization as a whole. Data Docs make it easy to work with stakeholders by automatically rendering business-ready visualizations and test results expressed in plain language. By using a common language, GX OSS frees you from translation efforts so you can focus on achieving mutual understanding.
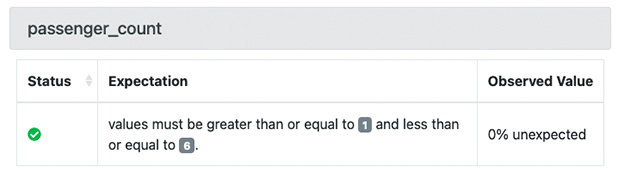
Take action
Your data systems never sleep, so your data quality processes can’t either. Take proactive action 24/7 with GX OSS: it integrates with your orchestrator to enable automation that ensures your data never goes unchecked. And by defining Actions alongside your Expectations, you can prevent bad data from entering a data source, keep it from moving downstream, notify relevant teams about critical failures, and more—all at the moment it’s detected.

Built on the strength of our ever-growing community
Our community is an inclusive space for data practitioners who want to improve data collaboration. With more than 11,000 data practitioners worldwide who have contributed to over 300 Expectations, our Slack community is the best place to get support from us and others working to maintain data quality at their organizations.
