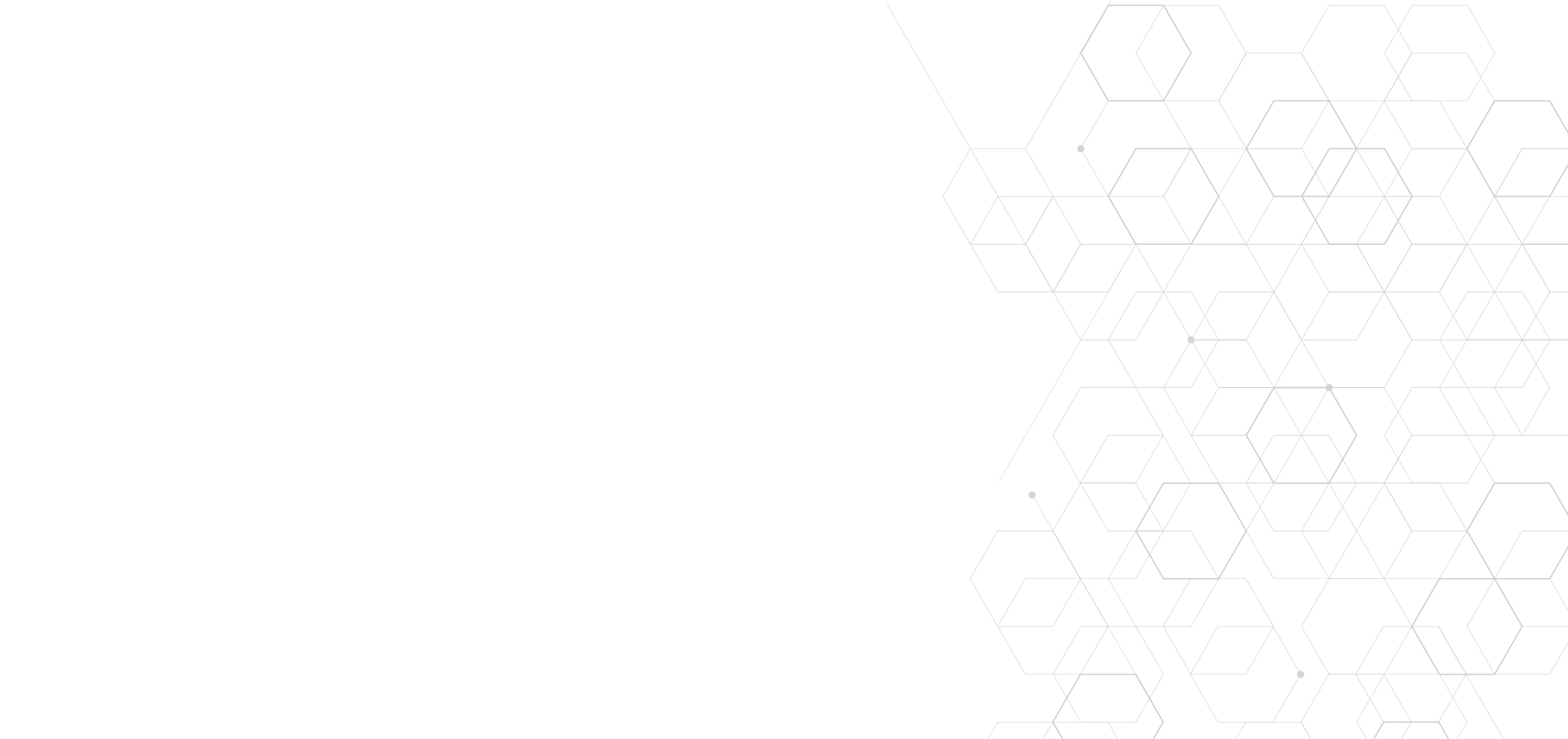
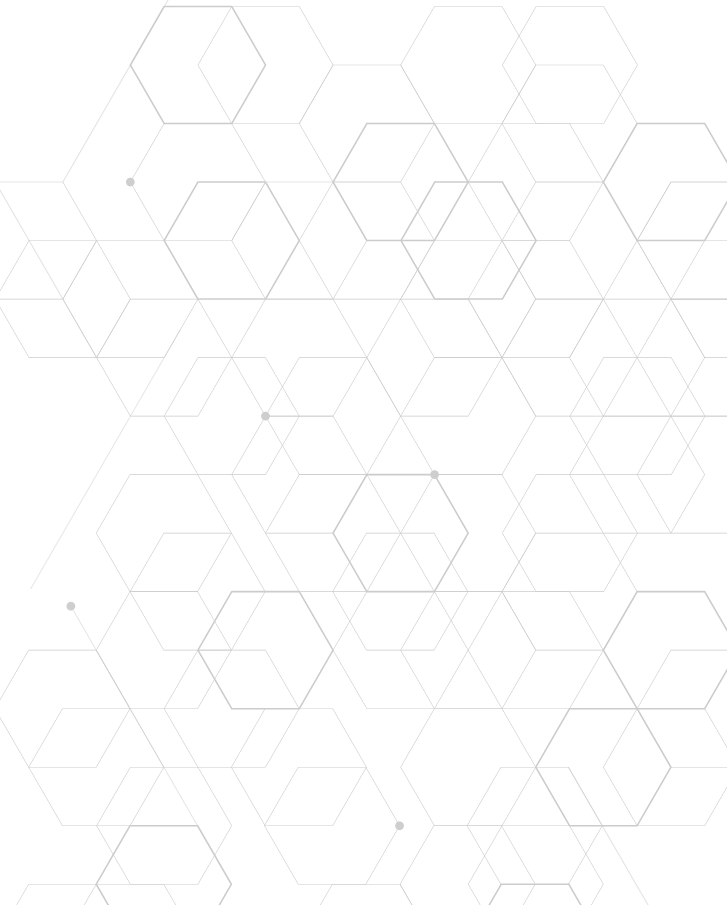
Great Expectations Case Study:
How Avanade uses GX to detect data drift from upstream model changes in machine learning pipelines
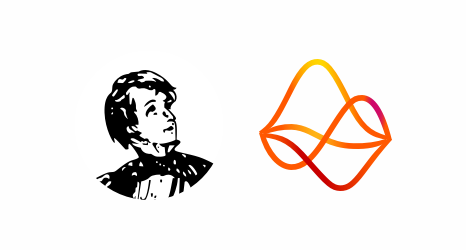
Scaffolding automatically profiles data to create initial Expectation Suite with manual cleanup, validation at every step of the pipeline
About Avanade
Avanade is a global professional services company providing IT consulting and services focused on the Microsoft platform. The company is a joint venture between Accenture and Microsoft, with 39,000 employees working in 25 countries . The main user of Great Expectations at Avanade, the Intelligent Enterprise Team, is located within the IT department, which focuses on servicing internal stakeholders with data insights into all relevant areas of the organization.
Avanade uses data to drive and support operational decisions. For that purpose, the IE team collects and uses data from various sources, such as sales applications, HR systems, and collaboration data from their Office 365 platform. The team works with all departments in the organization, ranging from sales, to finance, HR, and marketing, and provides stakeholders with a platform for unified data insights, reports, direct data access, and Data Science expertise.
The Challenge
One of the main challenges facing the Intelligent Enterprise Team when integrating data from so many distinct sources and departments is the frequent change of upstream data models and taxonomies, which were at risk of going unnoticed in their Machine Learning pipelines.
"Within our organization, we constantly run into taxonomy changes and business units that realign. We need to be able to know that so we can retrain our models, but we’re not always informed in advance."
Steve Nelson, Data Scientist at Avanade
One such issue was when the team noticed coincidentally that one of the top features feeding into their ML model had gone down to zero due to an issue "deep down" in the data warehouse, which would have severely impacted the model. Another example of data drift problems is the occurrence of outlier values that might have been introduced into the data as "dummy values" without the team noticing. The team evaluated another tool to identify feature drift, but decided that Great Expectations provided the most transparency for users to see what changed in their data, without hiding it behind opaque metrics. Another factor in that decision was the validation report output in Data Docs, which provides a convenient way to consume the validation output.
How the Avanade team uses Great Expectations
The Intelligent Enterprise Team relies on infrastructure based on a mix of Microsoft Azure cloud products and open source tooling, such as an on-prem SQL Server data warehouse, Azure Synapse, Azure Cloud Storage, Azure Data Factory, Azure ML Service, Power BI, Pandas, scikit-learn, and dbt.
To create Expectations, the team uses the scaffolding feature to automatically profile the data and create an initial version of an Expectation Suite, which is then cleaned up manually. They then validate the input data using those Expectation Suites in their Azure ML pipeline. Each step in the pipeline is followed by a validation step: First, the raw data is checked, and then the result of each transformation step is validated, too. The pipelines are configured to continue on validation failure, but they output an HTML table that contains an overview of which Expectations fail for which feature. The team is also planning to create a custom store for validation results, so that they can collect metrics on every validation run over time.
The Intelligent Enterprise Team reports that the biggest benefit of using Great Expectations has been the ability to catch data quality issues caused by upstream data changes before stakeholders notice.
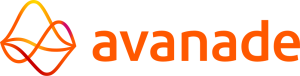
Integrated data from a variety of sources
Sales applications, HR systems, collaboration data from Office 365, and more
Insight into data drift
GX identifies feature drift and provides transparency to users
Ability to find issues caused by upstream data changes
GX helps catch data quality problems before stakeholders notice
Components
- Azure ML Service
- Power BI
- Pandas
- scikit-learn
- dbt