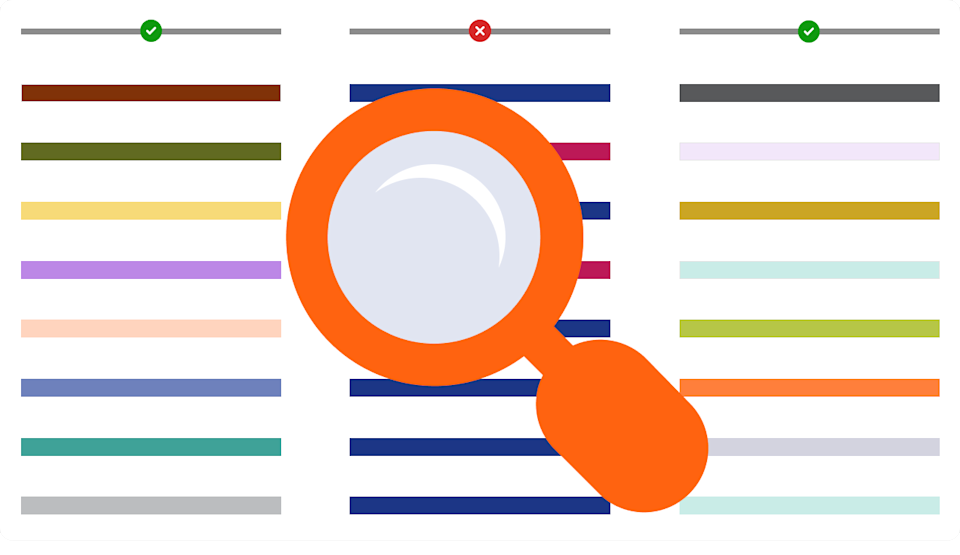
This post is the sixth in a seven-part series that uses a regulatory lens to explore how different dimensions of data quality can impact organizations—and how GX can help mitigate them. Read part 1 (schema), part 2 (missingness), part 3 (volume), part 4 (distribution), and part 5 (integrity).
Working with data can start to feel abstract, but much of your organization’s most critical data likely correspond to real, non-digital entities. Understanding when that’s the case and making sure that each real entity is ultimately represented an appropriate number of times—generally, once—in your dataset is an essential aspect of high-quality data.
Data uniqueness can empower organizations of all kinds to improve their efficiency, reduce costs, and innovate more effectively. But like all facets of data quality, it has its own challenges.
In this article, we’ll share a roadmap for excellence in understanding your data’s uniqueness.
Duplicate data: A silent business killer
Duplicate (aka non-unique) data can have negative side effects for organizations across industries. Here are some real-world scenarios that highlight the costs of duplicate data:
Revenue loss due to poor customer experiences. Duplicate customer records can lead to a whole host of negative experiences. For your organization, this includes misdirected communications, low-quality personalization and targeting, and incorrect billing. Meanwhile, customers can experience account and login problems, customer service issues, and difficulties with placing adn returning orders. The resulting erosion of customer trust and loyalty directly impacts your organization’s revenue and reputation.
Misguided decisions based on flawed business insights. Duplicate data can distort your analystics, leading to poor strategic choices. If sales figures are inflated by duplicate transactions, an ecommerce company will struggle to make accurate inventory decisions. If demographics are affected by duplicate customer records can cause an organization to misdirect their advertising.
Financial penalties and reputational damage from compliance and audit violations. Duplicate data can put companies at risk of non-compliance with regulations. In healthcare, duplicate patient records can lead to incorrect treatment decisions, which in turn can cause poor performance on core measures reporting—not to mention potential lawsuits. Duplicate transactions in a financial institution can raise red flags during audits, triggering costly investigations and potential fines.
Operational inefficiencies and increased costs. Managing and reconciling duplicate data takes up valuable time and resources. For example: sales teams can waste effort pursuing leads that have already been converted or lost. Customer service representatives may struggle to provide adequate support due to conflicting or missing information in available records. For healthcare providers, inaccurate or incomplete treatment and diagnosis information in patient records can cause suboptimal treatment recommendations and patient outcomes.
Missed opportunities for cross-selling and upselling. Capitalizing on your existing customer base is a critical part of any organization’s business strategy. Fragmented customer data can prevent you from identifying those valuable opportunities, resulting in lost revenue and decreased customer lifetime value.
Embark on your uniqueness journey
Having an excellent handle on your data’s uniqueness requires a systematic approach to implementing a proactive process. Here are the four steps you need to safeguard your data’s uniqueness and realize your best business outcomes:
Identify and prioritize critical data: Start with determining which data assets are most essential to business operations and decision-making, then collaborate stakeholders to define the uniqueness requirements—including the business rules that define what ‘unique’ means.
Implement uniqueness validation and deduplication: Using those business rules, develop uniqueness validation testing using a platform like GX, and automate the use of your uniqueness checks to ensure continuous monitoring. Then, use the results of your data validation to drive deduplication and record linkage efforts using approaches like deterministic and probabilistic matching.
Monitor, measure, and expand: As you continually monitor your data’s uniqueness in your data quality metrics, track the impact of your uniqueness validations. Quantify the business benefits of your improved data uniqueness to ensure that you’re taking meaningful actions. Then, iteratively expand your validation coverage and refine your deduplication process to further improve your outcomes.
Foster a culture of data quality ownership: Communicate the importance and benefits of monitoring your data’s uniqueness to stakeholders, and provide training and resources that help promote a shared responsibility for your data’s quality. Celebrate successes and share best practices throughout your organization. With a widespread culture of investment in data quality, you’ll be able to evolve your data quality process as your organization grows.
As we consistently recommend, using these steps allows data teams to start with focused, relatively simple efforts and progressively grow their efforts as their needs and resources allow. For technical information on implementing data uniqueness validation in GX, see our data uniqueness tutorial documentation.
Application: Lessons in assuring data uniqueness from FinTrust Bank
To explore how data uniqueness monitoring can look in a real-life application, we’ll continue our illustrative story of FinTrust Bank, a fictional financial institution, and their data team lead Samantha.
As FinTrust Bank increased data integration across departments, Samantha and her data team faced new challenges. The marketing department’s successful campaign—driven by data integrity improvements—produced a surge of new account openings and transactions, but inconsistencies began to emerge during data processing.
Investigation revealed that the issue stemmed from the data capture process. FinTrust Bank used multiple third-party vendors, who collected customer information in different data formations and used different validation rules. As FinTrust Bank integrated these different sources, the conflicts produced duplicate records in their main database. This non-unique data posed a significant risk to data integrity, with the potential to cause incorrect reporting and analysis.
Samantha and her team followed our four-step model in resolving the issue:
Samantha initiated a meeting with the marketing team to understand their data capture process and identify the critical fields where uniqueness was paramount.
The data team used GX to implement basic uniqueness validation and generate reports that identified duplicate and inconsistent records.
To tackle the identified duplicate records, the data team used a combination of matching techniques. First, they used deterministic matching based on unique identifiers—like SSNs and email addresses—to identify exact duplicate. Then then employed probabilistic matching using fuzzy logic on properties like names and addresses, to identify a further set of potential duplicates.
By reviewing the results and fine-tuning their matching thresholds, Samantha and her team were able to establish a reliable process to merge records into single customer views.Over the following months, Samantha’s team iterated on their uniqueness data quality testing to extend it into additional data assets. They also used their historical data quality metrics to quantify the business impact of their efforts.
Samantha communicated the positive outcomes of increased attention to data uniqueness throughout the company. This made it easier for her team to get resources from business stakeholders when continuing to expand their data quality monitoring.
With this structured approach, Samantha and her team were able to avert a duplicate data crisis at FinTrust Bank, and instead established a scalable framework for ensuring their data’s uniqueness.
For details on how uniqueness validations work in practice with GX, visit our technical documentation on validating data uniqueness.
Join the conversation
By prioritizing data uniqueness, you can gain a significant competitive edge over less-prepared competitors. The accurate, reliable, and trustworthy data that uniqueness monitoring helps ensure lets you make more informed decisions, better optimize your operations, and deliver personalized customer experiences that set your brand apart.
Data uniqueness is just one dimension of data quality that you need to consider as you stay ahead of the competitor. Visit the GX community forum to connect with like-minded professionals, share best practices, and discover innovative solutions.
Coming next: In our next post, we’ll discuss data freshness and how you can monitor your data’s timeliness across your organization.